Anomaly Detection in Time Series
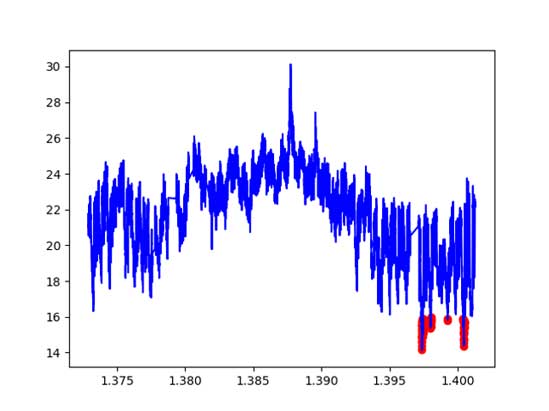
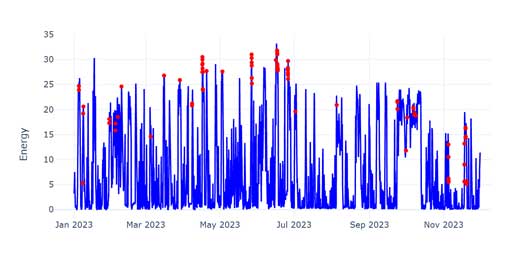
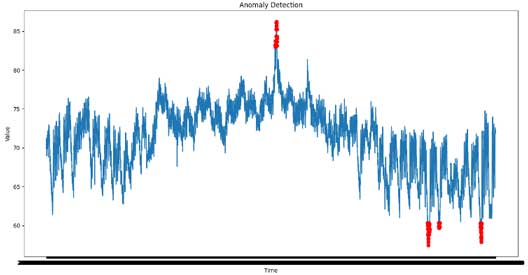
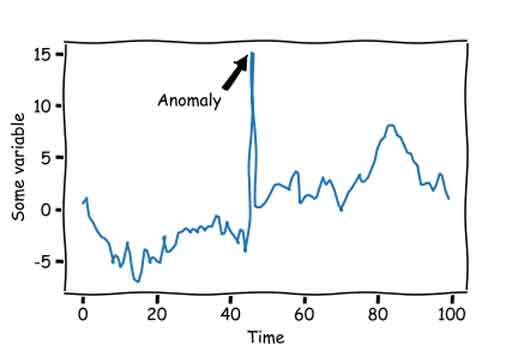
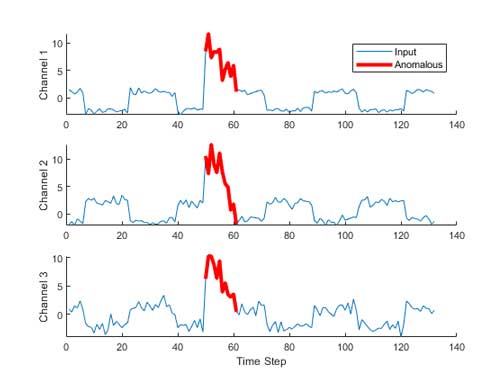
Anomalies are unusual data points (outliers) that deviate from common patterns in a dataset. In simple cases, deviations from the normal state are easily detectable, but as behavioral patterns and processes become more complex, accurate detection becomes increasingly challenging. These anomalies may indicate system errors, unexpected changes in processes, or even security threats. Detecting such points is particularly critical in areas such as cybersecurity, user behavior analysis, and industrial equipment monitoring.
Various methods have been developed for anomaly detection, ranging from classical statistical models to advanced deep learning algorithms. The main challenge in this field is distinguishing between noise and true anomalies while managing sparse and imbalanced data. Razintel’s anomaly detection service is capable of identifying different types of noise and detecting anomalies in time series data.
Razintel's Anomaly Detection Service
In today’s data-driven world, detecting anomalies in time series data is crucial for ensuring system reliability, security, and efficiency. Razintel’s anomaly detection service is designed to identify abnormal patterns in continuous, time-dependent data, enabling businesses and industries to proactively address potential risks.
Using a hybrid approach that combines neural networks, statistical methods, and deep learning, this service can accurately detect both simple and complex anomalies in time series data. Unlike traditional methods that struggle with noisy or imbalanced datasets, Razintel’s model effectively differentiates between normal fluctuations and genuine anomalies, ensuring precise detection.
This technology is particularly valuable in industrial equipment monitoring, where it can predict machinery failures before they occur, reducing downtime and maintenance costs. It also plays a critical role in financial system analysis, helping to detect fraudulent transactions, market irregularities, and operational inefficiencies. Additionally, in predictive maintenance, the system identifies early signs of degradation, allowing organizations to take preventative action before significant issues arise.
Tested on real-world industrial time series data, Razintel’s system has demonstrated exceptional performance in detecting both sudden anomalies (such as unexpected equipment failures) and long-term irregularities (such as gradual performance decline). Its real-time processing capabilities allow it to continuously analyze incoming data, providing instant alerts and actionable insights.
By integrating this advanced anomaly detection service, organizations can prevent unexpected failures, improve operational efficiency, and enhance decision-making through comprehensive analytical reports and intelligent dashboards. Whether in manufacturing, finance, healthcare, or IoT applications, Razintel provides a powerful, scalable solution for businesses looking to harness the power of time series anomaly detection.
KEY FEATURES INCLUDE
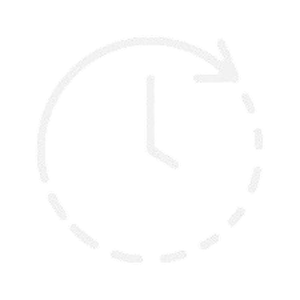
Real-time anomaly detection
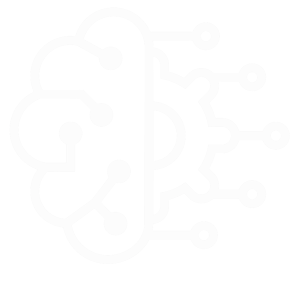
Adaptive machine learning models
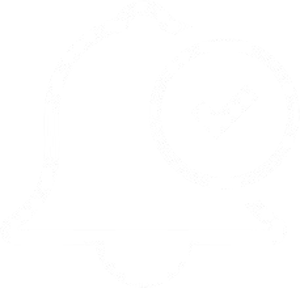
Customizable alerts & notifications
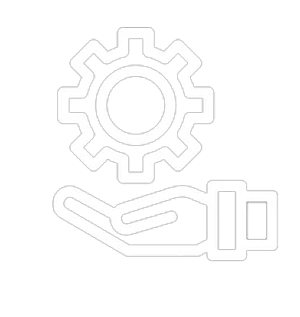
Multi-industry compatibility
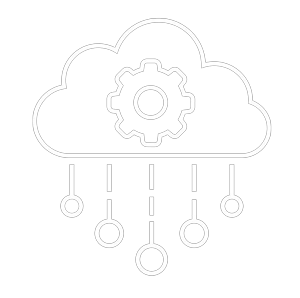
Scalable cloud-based solution
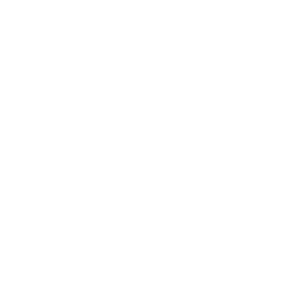
Comprehensive data visualization
FAQ
1- How accurate is this service?
The Razintel’s Anomaly Detection Service utilizes a combination of deep learning neural networks and statistical methods, enhancing accuracy in anomaly detection. While its performance varies depending on data type and operational conditions, testing on industrial equipment has demonstrated high precision in rapid anomaly detection and a significant reduction in false alarms. Additionally, its optimized algorithms can adapt to environmental changes, ensuring continuous performance improvement over time.
2- How can this service be deployed?
This service is designed for easy installation and deployment. Users can connect via API or a web-based dashboard to upload their time-series data seamlessly. It supports both on-premise installations (local server deployment) and cloud-based execution, offering flexibility based on organizational needs.
3- Does this service require a GPU?
This service can run on both CPU and GPU. Using a GPU significantly increases the processing speed of deep learning models. However, if users do not have access to a GPU, the system will still operate efficiently on standard processors with optimized performance.
4- What types of data does this service support?
This service is specifically designed for time-series data and can process behavioral patterns of industrial equipment, IoT sensors, financial data, and other time-based information. The data must be numerical and continuous and can be sent to the service in standard formats such as CSV, JSON, or via API.
5- What consideration has been taken to reduce false alarm rates?
To reduce false positives, a combination of neural networks and statistical methods has been implemented. This approach helps distinguish normal system variations from actual anomalies. Additionally, the system’s sensitivity can be adjusted, allowing users to control the strictness of anomaly detection.
6- Can the model be customized for specific applications?
Yes, this service allows model retraining with an organization’s specific data. Users can fine-tune the model based on their data and adjust parameters to meet their unique industrial or operational needs.
7- What is the system’s response time?
The Razintel’s Anomaly Detection Service is designed for real-time data processing. While response time depends on data volume and hardware specifications, tests have shown that the system can detect and report anomalies within seconds of their occurrence.
8- Can this service be integrated with other systems?
Yes, this service supports seamless integration via RESTful APIs, enabling connectivity with monitoring systems**, industrial platforms, and cloud services such as AWS, Google Cloud, and Azure. It is also compatible with various analytical software for enhanced data insights.